MANAGEMENT
MANAGEMENT
RESEARCH PAPER (ATTACHED)
2 pages, but not more than 3 pages, in the narrative and it should be typed in APA formatting (title page, reference page, no abstract page, double-spacing, Times New Roman 12 font, 1 inch margins, in-text citations, etc
T he word contingency has been given a completely new meaning since it was introduced to heavy oil and gas energy construction industry. Perhaps it is one of the most confusing concepts in project cost and
schedule management systems. More often than not, the contingencies are simply hidden in the base estimate, causing chaotic results [7]. Now, with the historical cost data becoming illusory, the efforts of benchmarking will be misleading and meaningless. Lack of structured transparency has led to the misconception that the contingency fund is a phenomenon of wide utility as a catch-all to cover project extra costs which otherwise can not be legitimately accounted for.
J.P. Morgan has been quoted as saying that “the market will fluctuate” [2]. This claim also applies to project cost forecasts. Contingency forecasts fluctuate as well, following the project progress and overall project risk shifts. One of the most effective ways to avoid the catch-all syndrome is to break down contingency into risk affected components. The level of detail shall be driven by a well established work breakdown structure (WBS), which shall be inherently built into a contingency risk model. When the contingency amount is derived, it will be automatically dispersed into appropriate accounts based on the risk levels and cost weigh- ing factors.
Allocating contingency instead of a single liner provides a great advantage to the management of the contingency. By using moderate cost breakdown details, the cost engineer knows exactly how the contingency model is put together and the amount each account deserves, and the timing when the amount is exhausted. Hence, the cost engineer can easily generate a contingency draw- down plan, and manage the depletion of contingency in a controlled manner.
PROJECT COST OVERRUN PHENOMENON
Fifteen years after the economic recession of the 1990s—a result of a world-wide oil price drop, the Oil Sands in northern Alberta of Canada became a hot spot, attracting numerous investors from the United States and other parts of the world. Scarcity of oil and its current high price were the catalysts for a booming oil and gas construction industry in the province of Alberta. Between 2005 and 2013, a total amount of 63.5 Billion
Canadian dollars [6] will be invested in the exploration, mining, crude refining and transportation of bituminous oil sands. However, the heavy construction in the energy industry has been plagued with a history of poor performance, budget overrun and significant schedule delays, according to the Construction Owner’s Association of Alberta (COAA).
Various attempts have been made by the COAA and other interest groups to improve the reputation of the industry with little success to date. Perhaps there is no easy way to save the industry’s notoriety for cost overruns. However, one method to avoid further cost slippage—the implementation of a stringent risk management process, including adequate cost and schedule contingency—is being advocated by the author. According to Dr. J. Murther , a Houston-based risk management consultant, the reason that risk-based contingency management has not yet been overwhelmingly successful in the energy construction sector is because the project risk management is still in its infant cradle and not yet fully embraced by the owners’ senior management.
Although contingency has become a buzz word, it is unfortunately not being truly understood in the heavy oil and gas construction industry. In fact, the term contingency fund has been widely misused—for project scope creep or to cover extra project costs which have otherwise been either omitted from the estimate or have arisen as a surprise.
It is also not uncommon for the contingency fund to be kept as a secret in many projects. Hartman [7] highlighted this problem in his assertion that “hidden contingencies are hard to manage” and that “[getting] them in the open without having them taken away” poses a significant challenge, not only to the project management team but also – and more importantly—to the risk analyst.
CONFUSION AND MISCONCEPTIONS ABOUT CONTINGENCY
Risk and uncertainty are inherent to projects during their life cycles. Stralser [16] noted that “not many project managers realize that managing risk is their primary responsibility.” Project managers make decisions, give directions, select optimal options and spend large amounts of money, often without realizing that they are managing various risks; they are going through a rigorous
RISK.05.1
2006 AACE International Transactions
RISK.05
Significance of WBS in Contingency Modeling
Mr. John Gengxin Zhao
risk management and decision-making process. However, not all risks can be effectively managed to an acceptable level, and sometimes “risk taking is a major aspect of managerial work” [15], with contingency being one of the most popular forms of project risk management [7].
Capital cost estimate is to some extent a perfect example of human error, estimate preparation timing, quality of input data and completeness of design, maturity of engineering deliverables and project contracting strategy, as well as the execution plan – all predominate their risky states and uncertain conditions. While some still refuse to acknowledge the factual characteristics of the cost estimate, many are prepared to take the risks, but have applied for an adequate contingency as a safeguard; “contingency has been added to enable us to expect the unexpected” [21]. Clark and Lorenzoni [5] suggest that a “technology of contingency” be used to cover these risks and uncertainties since the perfection and 100 percent accuracy of a capital cost estimate will never be achievable due to its inherent uncertainties and the nature of the construction industry.
When project managers make decisions, they are faced with three situations: certainty, risk, and uncertainty [15]. Risky and uncertain situations empower probability-based decision-making techniques. A contingency fund, often derived from Monte Carlo simulation, is consequently added to improve the managers’ confidence level and aid a better and quicker decision-making process.
However, it is this very covert contingency fund that has caused many misconceptions, ad hoc interpretations, and confusion in the construction industry. Worse yet, some overconfident project managers completely ignore the use of contingency, assuming that they have a perfect cost estimate that they can maintain until the end of the project completion: “Unnecessary doubts may incapacitate the professional, and create an impression of incompetence, but unjustified complacency can be equally disastrous in the long run” [18]. On the contrary, some project managers opt to hide the contingency fund without any transparency to the project team, leaving the project struggling with an inadequate budget from the onset. At best, the contingency is calculated and managed at a higher level—normally just an entry or a single line item in the cost management system—leaving project team members to wonder what this contingency actually entails.
Eventually all that is desired is to cost effectively manage project risks by improving the project uncertainties in order to eliminate or reduce the chances of project failure.
THE IMPORTANCE OF WORK BREAKDOWN STRUCTURE
Beholding the current industry situation, one way to better understand and manage the project contingency is to break the contingency fund down and allocate it into various slots based on the work breakdown structure (WBS). The WBS “turns one large, unique, perhaps mystifying, piece of work, project, into many small manageable tasks” [17]. In conjunction with the WBS, a new taxonomy, risk breakdown structure (RBS), has also been used for better risk and contingency management using the same
WBS principles. While the WBS helps provide visibility to important or risky work efforts [4], the RBS can assist in understanding the distribution of risk on a project or across a business, aiding effective risk management [10].
In discussing the work breakdown structure, Hillson [11] stated: “The most obvious demonstration of the value of structur- ing within project management is the work breakdown structure (WBS), which is recognized as a major tool for the project manager because it provides a mean to structure the work to be done to accomplish project objectives.”
The importance of the WBS is further emphasized by Verzuh [17] who states that “it is the foundation of project planning and one of the most important techniques used in project management.” With the help of the WBS, the exorbitant cost estimate or project schedule can be broken down into meaningful, manageable and traceable pieces, not only for the purpose of quantitative cost/schedule risk analyses but also for cost management and schedule updating.
In the qualitative risk assessment process, the risk breakdown structure (RBS) offered “a source-oriented grouping of project risks that organizes and defines the total risk exposure of the project” in a hierarchical structure [11]. Currently, the use of the RBS is limited to risk identification and ranking and reporting processes, although it has the potential to become the most valuable single tool in assisting the project manager to understand and manage risks to his project in a quantitative manner. Despite the fact that many individuals, as well as corporations, have attempted to produce an integrated RBS based WBS using emerging technology—such as London-based Pertmaster™ and US-based Ares Corporation’s PRISM Risk Manager™—to aid quantitative risk assessment, the true benefits of this integrated approach have yet to be tested, realized and ascertained.
When building a risk model to simulate cost estimate or schedule contingency using the Monte Carlo technique, proper breakdown of the estimate or schedule to leverage the contents of variables in each WBS account is always a difficult task. Each WBS account bears different types and degrees of risks; therefore the variability of uncertainty can be a wide swing between accounts. An unbalanced WBS could severely skew the simulation results because the variations of risks could be generalized instead of specific. A set of calibrated WBS with the help of RBS inputs will significantly enhance the reliability of the contingency model.
WBS INHERENT RISK MODEL TECHNIQUE
Statistics work only for large numbers [2], and when a large amount of variables are assessed in a model, the Monte Carlo simulation tends to produce a symmetrical bell-curve probability density function (PDF) in the shape of a histogram, according to Laplace’s (1809) Central Limit Theorem. These large volumes of variables appear and poise in a very disorderly pattern, which makes data management a nightmare. Further more, the task of systematically assigning uncertainty ranges to inter-related variables without a structured data breakdown framework not only becomes cumbersome and time consuming, but also looses consistency and train of thinking process.
RISK.05.2
2006 AACE International Transactions
“Order is impossible to find unless disorder is there first” [2]. The use of the Monte Carlo simulation aims to better forecast project future outcomes—a process which involves a tremendous amount of quantitative data in a disorderly manner. Since the Monte Carlo simulation is a type of parametric simulation, little historical data or benchmark information is known [13]. Mun [13] also stressed that “forecasting is the art and science of predicting the future. It can be based on quantitative approach and qualitative approach.” This qualitative approach largely depends upon expert judgement and management assumptions. Without a WBS aided thinking process, the human brain is simply not capable of effectively handling more than seven pieces of data, according to psychologists (Greenwood, 2004).
A typical $500 million cost estimate can easily contain between 200—300 variables, of which more than 50 percent need to be carefully assessed to derive a meaningful estimate contingency as the result of acceptance of the risky state and conditions of the cost estimate. For instance, the validity of a single point cost estimate at EDS stage, when only approximate 50 percent engineering is progressed, relies on the entry of contingency [9] to achieve the required confidence level. Future predictability of the true estimate outcome depends on many variables. Even with those variables achieving some degrees of certainties, the potential changes during the course of execution and human errors during the estimate preparation will ultimately alter the final estimate results. Using the WBS to help break down
the estimate—figure 1— has far reaching effects for both cost management and contingency depletion, because:
• Future changes can be attributable to the associated WBS accounts;
• Human errors can be traceable back to appropriate WBS home domains;
• Responsible individuals can be accountable for respective WBS contingency;
• Potential savings can be recognizable in corresponding risk- free WBS.
Intuitive judgement based on heuristics and biases [12] plays an important role in deciding which variables are to be analyzed. Past experiences have accumulated dense mindsets among engineering and construction professionals in regards to assessing risks. Variables that are often surfaced for evaluations have been anchored in their minds , while hidden and invisible variables are often neglected or ignored. Without the help of a structured approach, risk analysis is merely an activity of the random selection of apparent variables for ranging exercises. This phenomenon was also discovered during the author’s experiences of 22 risk workshops and nine major quantitative estimate and schedule assessments in various oil and gas energy projects.
Regression correlations among those variables can drastically skew the final statistical results. Correct and righteous variable dependency requires that the implementation and application of
RISK.05.3
2006 AACE International Transactions
Figure 1—WBS Based Cost Elements
Figure 2—WBS Based Labour Cost Risk Model
the WBS be built in the simulation models. The following examples of typical correlations illustrate the criticality of the existence of a WBS in cost risk simulation modelling.
Positive correlation If the Quantity of Piping account Materials Take-Off [WBS:
1-3.02-Q] is increased, the labour manhours for the same Piping account [WBS: 1-3.02-L] will be positively increased by a percentage (say 60 percent). EHT and insulation subcontractor [WBS: 1-6-03-S] pricing will also be increased, positively, by a percentage (say 35 percent). Indirect and construction management costs [WBS: 2-11.04-O] are also slightly increased by 10 percent.
Negative correlation The example of the negative correlation is that if the shop
modularization quantity is increased, the field labour manhours for structural steel and piping accounts will be decreased, and field indirect costs and camp usage costs will also be decreased.
“Analyzing a system can not be selective process, subject to a single perspective of an analyst” [22]. A risk simulation model without a structured hierarchy and the sorting ability to differentiate various risk components may easily experience this pitfall. Preferences for selective and apparent variables will not only generate unreliable statistical results but also an incomplete and unsuccessful model. Inconsistent simulation results, which are based on variable preferences, will also not be beneficial for the purpose of meaningful benchmarking. Only a preformatted analytical simulation conclusion based on a pre-established WBS will help to avoid this pitfall and achieve correct statistics.
CONCLUSION: PRE-FORMATTED WBS ENHANCING MODELLING RESULTS
To avoid “applying a politically correct percentage” to the number, a more intelligent means of adding contingency [7] is to use the Monte Carlo simulation technique by engaging all stakeholders in the game play. Doing so demands the colossal cost estimate be dismantled and sorted structurally.
Heinze [9] stresses the importance that “a major project is broken down into various cost components first.” The Monte Carlo simulation model mimics the real project scenario, depicting the estimate and cost control system structure. Typically, the model is built around an organization’s WBS and tweaked with an RBS to reflect its risk tolerability, and also for the decision making process. In the model, each variable is represented by a WBS/RBS code residing in its designated domain, and the variability of its upper and lower limits, pessimistic and optimistic views, is measured and endorsed collectively by a group judgement, or by using Delphi Technique.
The importance of a WBS-based risk analysis approach was strongly advocated by David Vose [20]. In his book “Risk Analysis: A Quantitative Guide,” he says of the cost risk analysis: “A cost risk analysis is usually developed from a work breakdown structure (WBS) which is a document that details, from the top down, the various work packages (WP) of which the project consists.”
Structured risk assessment of variables minimizes the risk of omitting critical items; it systematically scrutinizes the uncertainties of MTO quantity, material cost, wage rate, productivity factor (PF) and estimated man-hours. See figure 2. When an incurred cost is confirmed, it can be easily removed from the estimate following its WBS domain, because risk management is the ability to define what may happen in the future [1].
T he whole purpose of modelling risks goes to the heart of cost management: cost effectively manage risks by depleting contingency. Appropriate allocation of the contingency funds to risk-affected WBS accounts also
increases the project manager’s accountability following the risk allocation principle [3]. Further more, the contingency, derived from the simulation process, must be properly scaled and dispersed into WBS accounts following the approved project execution schedule; this dispensation of contingency funds over time is referred to as contingency drawdown management plan.
“All contingency budgets should be tied to specific risks” [19]. The advantages of locking contingency into WBS based risk accounts not only provides project managers with certain flexibilities in managing their cost forecasts, but also prevents
RISK.05.4
2006 AACE International Transactions
Figure 3—WBS Based Risk Simulation Results
borrowing contingency money from other accounts or advancing from future timeline.
The catch-all syndrome is eliminated and details of contingency are transparent. The visual sensation of the adequacy of the remaining contingency against the remaining work and risk levels, depicted in a draw-down curve , clearly illustrates the characteristics of the project manager’s risk attitude, i.e. risk aversion or risk taking.
“A risk analysis model, however carefully crafted, is of no value unless its results are understandable, useful and believable” [20]. The original estimate amount in comparison with the simulated total amount, including contingency by WBS accounts—figure 2—provides the project management team with a clear and direct pictorial understanding of the contingency modelling technique. It is of equal value to estimators, cost analysts, project executors and contract administrators as well as project managers. Subsequently, a WBS-based risk analysis and contingency modelling technique add net value to the project team.
REFERENCES
1. Aven, Terje (2003) Foundations of Risk Analysis – A knowledge and decision oriented perspective. University of Stavanger, Norway. John Wiley & Sons Ltd. West Susses, England.
2. Bernstein, Peter (1996) Against The Gods – The Remarkable Story of Risk. John Wiley & Sons, Inc. New York, USA.
3. Chapman, Chris and Ward, Stephen (2003) Project Risk Management – Processes, techniques and Insights. University of Southampton. John Wiley & Sons, Ltd. Sussex, England
4. Chapman, James (2005) Principle based Project Management. Washington DC. Accessed 26 November 2005. < http://www.hyperthot.com/pm_wbs.htm>
5. Clark, Forrest D and Lorenzoni, A.B. (1997) Applied Cost Engineering, 3rd Edition. New York, Marcel Dekker Inc.
6. Global and Mail (2005) Alberta Oil Sands Development, Business Section, December 4, 2005, Calgary Newspaper, Alberta, Canada.
7. Hartman, Francis (1999) Don’t Park Your Brain Outside: A practical guide to improving shareholder value with SMART Management. Pennsylvania, USA, Published by Project Management Institute Inc.
8. Harvard Business Essentials (2004) Crisis Management – Master the Skills to Prevent Disasters. Harvard Business School Publishing, Boston, USA.
9. Heinz, Kurt (1996) Cost Management of Capital Projects. Marcel Dekker, Inc. New York, USA.
10. Hillson, David (2005) Using a Risk Breakdown Structure in Project Management. Accessed 27 November 2005. <http://www.apmg-icp.com/request158.apmg >
11. Hillson, David (2002) Using a Risk Breakdown to Understand your Risks. Texas, USA, Project Management Professional Solutions Ltd. Accessed 27 November 2005. <http://www.risk-doctor.com/pdf-files/rbs1002.pdf >
12. Kahneman, Daniel, Tversky, Amos and Slovic, Paul (1982) Judgment Under Uncertainty: Heuristics and Biases. Cambridge University Press, Cambridge, UK.
13. Mun, Johnathan, (2004) Applied Risk Analysis – Moving Beyond Uncertainty in Business. John Wiley & Sons, Inc. New Jersey, USA.
14. Purba, Sanjiv and Zucchero, Joseph (2004) Project Rescue – Avoiding a Project Management Disaster. McGraw- Hill/Osborne, 2004.
15. Shapira, Zur (1995) Risk Taking: A managerial perspective. New York, published by Russell Sage Foundation
16. Stralser, Steven (2004) MBA in A Day. John Wiley & Sons. Inc. New Jersey, US.
17. Verzuh, Eric (1999) The Fast Forward MBA in Project Management. John Wiley & Sons, Inc. New York, USA.
18. Veryard Projects (2004) Contingency and Risk Models. Accessed 1 December 2005. <http://www.veryard.com>
19. Veryard Projects (2005) Three Notions of Contingency. Accessed 16 December 2005 <http://www.users.globalnet.co.uk/~rxv/projmgt/contin- gency.htm>
20. Vose, David (2000) Risk Analysis – A Quantitative Guide, John Wiley & Sons. Ltd. West Sussex, England.
21. Whitten, Neal (2005) No-nonsense advice for successful proj- ects. Vienna, VA, Published by Management Concept Inc.
22. Yacov Y. Haimes (2004) Risk Modelling, Assessment, and Management.
John Wiley & Sons. Inc. New Jersey, USA. 23. Berger, Bob (1994) Beating Murphy’s Law. Dell Publishing,
Yew York, USA. 24. Drucker, Peter (2003) On the Profession of Management.
Harvard Business School Publishing, Boston, MA, USA 25. Jorion, Philippe (1995) Value at Risk. The McGraw-Hill
Companies, Inc. USA. 26. Finkel, Adam M (1990) Confronting Uncertainty in Risk
Management. Centre for Risk Management, resources for the Future, January 1990, Washington D.C.
Mr. John Gengzin Zhao Senior Cost Engineer
Suncor Energy Services, Inc. PO Box 38, 112-4th Avenue, SW
Calgary, AB T2P 2V5 Canada Phone: 403-920-8576
Email: jzhao@suncor.com
RISK.05.5
2006 AACE International Transactions
"Looking for a Similar Assignment? Get Expert Help at an Amazing Discount!"
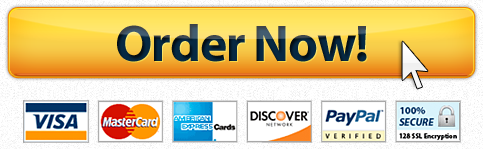